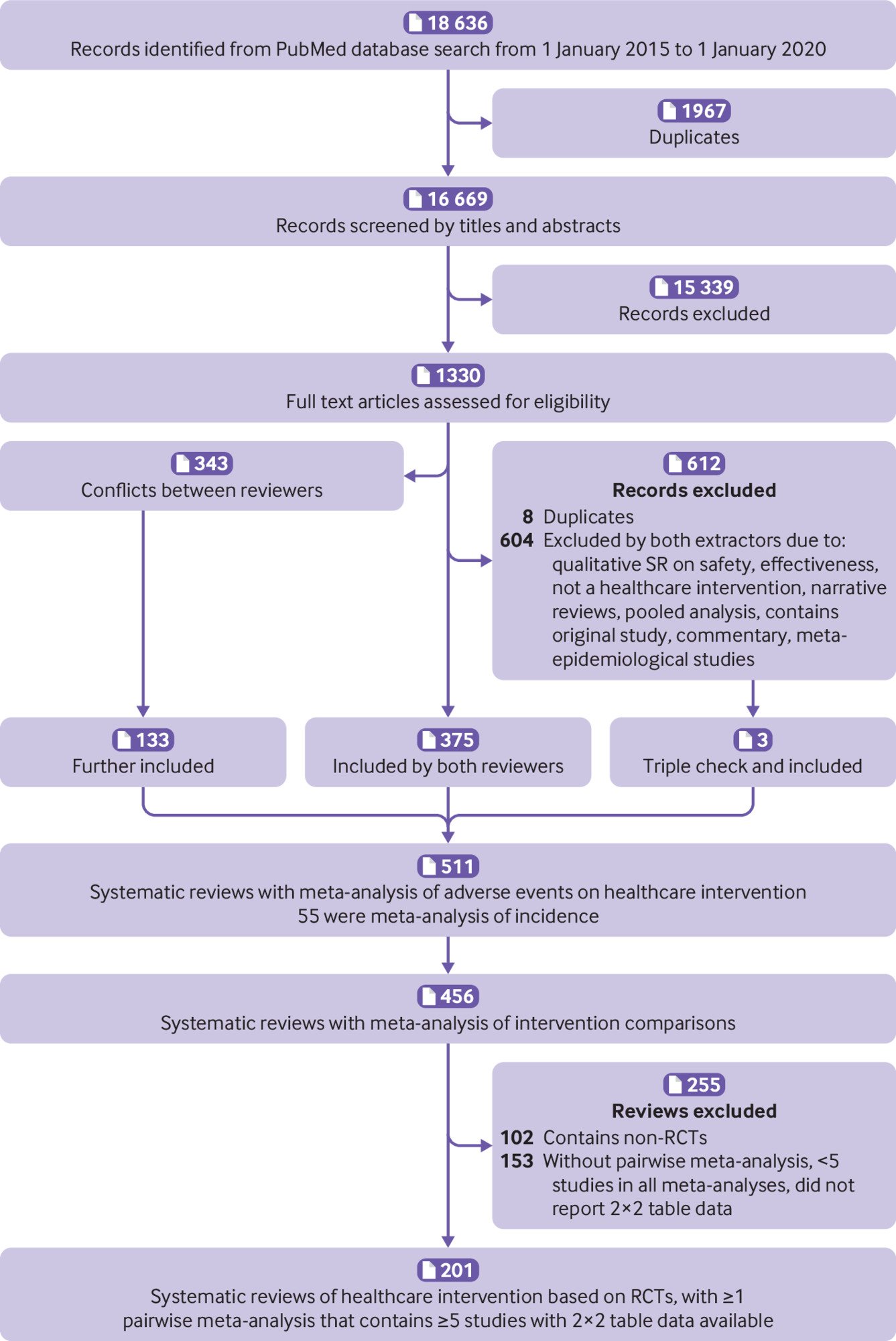
Abstract
Objectives To investigate the validity of data extraction in systematic reviews of adverse events, the effect of data extraction errors on the results, and to develop a classification framework for data extraction errors to support further methodological research.
Design Reproducibility study.
Data sources PubMed was searched for eligible systematic reviews published between 1 January 2015 and 1 January 2020. Metadata from the randomised controlled trials were extracted from the systematic reviews by four authors. The original data sources (eg, full text and ClinicalTrials.gov) were then referred to by the same authors to reproduce the data used in these meta-analyses.
Eligibility criteria for selecting studies Systematic reviews were included when based on randomised controlled trials for healthcare interventions that reported safety as the exclusive outcome, with at least one pair meta-analysis that included five or more randomised controlled trials and with a 2×2 table of data for event counts and sample sizes in intervention and control arms available for each trial in the meta-analysis.
Main outcome measures The primary outcome was data extraction errors summarised at three levels: study level, meta-analysis level, and systematic review level. The potential effect of such errors on the results was further investigated.
Results 201 systematic reviews and 829 pairwise meta-analyses involving 10 386 randomised controlled trials were included. Data extraction could not be reproduced in 1762 (17.0%) of 10 386 trials. In 554 (66.8%) of 829 meta-analyses, at least one randomised controlled trial had data extraction errors; 171 (85.1%) of 201 systematic reviews had at least one meta-analysis with data extraction errors. The most common types of data extraction errors were numerical errors (49.2%, 867/1762) and ambiguous errors (29.9%, 526/1762), mainly caused by ambiguous definitions of the outcomes. These categories were followed by three others: zero assumption errors, misidentification, and mismatching errors. The impact of these errors were analysed on 288 meta-analyses. Data extraction errors led to 10 (3.5%) of 288 meta-analyses changing the direction of the effect and 19 (6.6%) of 288 meta-analyses changing the significance of the P value. Meta-analyses that had two or more different types of errors were more susceptible to these changes than those with only one type of error (for moderate changes, 11 (28.2%) of 39 v 26 (10.4%) 249, P=0.002; for large changes, 5 (12.8%) of 39 v 8 (3.2%) of 249, P=0.01).
Conclusion Systematic reviews of adverse events potentially have serious issues in terms of the reproducibility of the data extraction, and these errors can mislead the conclusions. Implementation guidelines are urgently required to help authors of future systematic reviews improve the validity of data extraction.
Introduction
In an online survey of 1576 researchers by Nature, the collected opinions emphasised the need for better reproducibility in research: “More than 70% of researchers have tried and failed to reproduce another scientist’s experiments, and more than half have failed to reproduce their own experiments.”1
Systematic reviews and meta-analyses have become the most important tools for assessing healthcare interventions. This research involves explicit and standardised procedures to identify, appraise, and synthesise all available evidence within a specific topic.2 During the process of systematic reviews, each step matters, and any errors could affect the reliability of the final results. Among these steps, data extraction is arguably one of the most important and is prone to errors because raw data are transferred from original studies into the systematic review that serves as the basis for evidence synthesis.
To ensure the quality of data extraction, authoritative guidelines, such as the Cochrane Handbook, highlight the importance of independent extraction by two review authors.2 Despite this quality assurance mechanism, data extraction error in systematic reviews occurs frequently in the literature.3 Jones et al4 reproduced 34 Cochrane reviews published in 2003 (issue 4) and found that 20 (59%) had data extraction errors. Gøtzsche et al5 examined 27 meta-analyses of continuous outcomes and reported that 17 (63%) of these meta-analyses had an error for at least one of the two randomly selected trials. In their subsequent study, based on 10 systematic reviews of continuous outcomes, seven (70%) were identified as erroneous data.6
Empirical evidence suggests that the effect of data extraction error seems to be minor.35 However, this conclusion is based on systematic reviews of continuous outcomes, which do not apply to binary outcomes of adverse events. Harms, especially serious harms, tend to be rare, and such data in nature are more susceptible to random or systematic errors than are common outcomes.78 For example, consider a 1:1 designed trial with a sample size of 100, and the event counts of death are two intervention group and one in the control group. If the review authors incorrectly extracted the number of events in the intervention group as one, the relative risk would drop from two to one, leading to a completely different conclusion. Owing to this feature, in systematic reviews of adverse events, the validity of data extraction can considerably affect the results and even predominate the final conclusion. The erroneous conclusion would further influence the clinical practice guidelines and mislead healthcare practice.
We used a large-scale reproducibility investigation on the reproducibility of data extraction for systematic reviews of adverse events. We propose an empirical classification of the data extraction errors to help methodologists and systematic review authors better understand the sources of data extraction errors. The impact of such errors on the results is also examined based on the reproducibility dataset.
Methods
Protocol and data source
This article is an extension of our previous work describing methods to deal with double-zero-event studies.9 A protocol was drafted on 11 April 2021 by a group of core authors (CX, TY, LL, LFK), which was then revised after expert feedback (SV, LZ, RQ, and JZ; see supplementary file). We also record the detailed implementation of this study (supplementary table 1).
A subset of the data from the previous study was used in this study. Briefly, we searched PubMed for systematic reviews of adverse events indexed from 1 January 2015 to 1 January 2020. The limit on the search date was arbitrary but allowed us to capture the practice of the most recent systematic reviews. We did not search in other databases because we did not aim to include all systematic reviews; instead, a representative sample was sufficient for the aim of the current study. The search strategy was developed by an information specialist (supplementary box 1), and the literature search was conducted on 28 July 2020, and has been recorded elsewhere.9
Inclusion criteria and screening
We included systematic reviews of randomised controlled trials for healthcare interventions, with adverse events as the exclusive outcome. The term adverse event was defined as “any untoward medical occurrence in a patient or subject in clinical practice,”10 which could be a side effect, adverse effect, adverse reaction, harm, or complication associated with any healthcare intervention.11 We did not consider systematic reviews based on other types of studies because randomised controlled trials are more likely to be registered with available related summarised data for safety outcomes; this source provided another valid way to assess the reproducibility of data extraction. Additionally, we limited systematic reviews to those with at least one pairwise meta-analysis with five or more studies; the requirement of the number of studies was designed for an ongoing series of studies on synthesis methods to ensure sufficient statistical power.12 To facilitate the reproducing of the data used in meta-analyses, we considered only systematic reviews that provided a 2×2 table of data of event counts and sample sizes in intervention and control arms of each included study in forest plots or tables. Meta-analyses of proportions and network meta-analyses were not considered. Safety outcomes with continuous type were also not considered because continuous outcomes have been investigated by others.456 Systematic reviews in languages other than English and Chinese were excluded.
Two review authors screened the literature independently (XQ and CX). Titles and abstracts were screened first, and then the full texts of the relevant publications were read. For screening of titles and abstracts, only records excluded by both reviewer authors were excluded. Any disagreements were solved by discussion between the two authors.
Data collection
Metadata from the randomised controlled trials were collected from eligible systematic reviews. The following items were extracted: name of the first author, outcome of interest, number of participants and number of events in each group, and detailed information of intervention (eg, type of intervention, dosage, and duration) and control groups. Four experienced authors (CX, TQ, XQ, and HM) extracted the data by dividing the eligible systematic reviews into four equal portions by the initial of the first author, and each extractor led one portion. We had a pilot training for the above items to be extracted through the first systematic review before the formal data extraction. Finally, data were initially checked by the same extractors for their own portion and double checked by the other two authors separately (CX and TQ) to confirm that no errors were present from the data extraction (supplementary table 1).
Additionally, based on the reporting of each systematic review, we collected the following information according to the good practice guideline of data extraction13: how the data were extracted (eg, two extractors independently), whether a protocol was available, whether a clear data extraction plan was made in the protocol, whether any solution for anticipant problems in data extraction was outlined in the protocol, whether a standard data extraction form was used, whether the data extraction form was piloted, whether the data extractors were trained, the expertise of the data extractors, and whether they documented any details of data extraction. CX also collected the methods (eg, inverse variance, fixed effect model), effect estimators used for meta-analysis, and the effect with a confidence interval of the meta-analysis. TQ checked the extraction and any disagreements were solved by discussion between these two authors (with detailed records).
Reproducibility
After we extracted the data from the included systematic reviews, the four authors who extracted the data were required to reproduce the data used in meta-analyses from the original sources, which included the original publications of the randomised controlled trials and their supplementary files, ClinicalTrials.gov, and websites of the pharmaceutical companies. When the trial data used in a meta-analysis were not the same as had been reported from one of its original sources, we classified it as a “data extraction error.” If the authors of the systematic review reported that they had contacted the authors of the original paper and successfully obtained related data, we did not consider the discrepancy a data extraction error, even if the data were not the same as any of the original sources.14 We recorded the details of the location (that is, event count (r) or total sample size (n), intervention (1) or control group (2), which are marked as r1/n1/r2/n2) and the reasons why the data could not be reproduced. Any enquires or issues that would affect our assessment were resolved by group discussion of the four extractors. Again, reproducibility was initially checked by the data extractors for their own portions of the workload. After data extraction and reproduction, the lead author (CX) and TQ separately conducted two further rounds of double checking (supplementary table 1).15
Outcomes
Our primary outcome of this study was the proportions of the data extraction errors at the study level, the meta-analysis level, and the systematic review level. The secondary outcomes were the proportion of studies with data extraction error within each meta-analysis and the proportion of meta-analyses with data extraction error within each systematic review.
Statistical analysis
We summarised the frequency of data extraction errors at the study level, the meta-analysis level, and the systematic review level to estimate the aforementioned proportions. For the study level, the frequency was the total number of randomised controlled trials with data extraction errors. For the meta-analysis level, the frequency was the number of meta-analyses with at least one study with data extraction errors. For the systematic review level, the frequency was the number of systematic reviews with at least one meta-analysis with data extraction errors.
Considering that clustering effects might be present (owing to the diverse expertise and experience of the four people who extracted data), a generalised linear mixed model was further used to estimate the extractor adjusted proportion.16 The potential associations among duplicated data extraction, development of a protocol in advance, and data extraction errors based on systematic review level were examined using multivariable logistic regression. Other recommendations listed in good practice guidelines were not examined because most systematic reviews did not report the information.
Because data extraction errors could have different mechanisms (eg, calculation errors and unclear definition of the outcome), we empirically classified these errors into different types on the basis of consensus after summarising the error information (supplementary fig 1). Then, the percentages of the different types of errors among the total number of errors were summarised based on the study level. We conducted a post-hoc comparison of the difference of the proportions of the total and the subtype errors by two types of interventions: drug interventions; and non-drug interventions (eg, surgery and device). We did this because the safety outcomes are greatly different for these two types of interventions based on our word cloud analysis (supplementary fig 2).
To investigate the potential effect of data extraction errors on the results, we used the same methods and effect estimators that the authors reported based on the corrected dataset. We repeated these meta-analyses and compared the new results to the original results. Some meta-analyses contained errors related to unclear definitions of the outcomes (that is, the ambiguous error defined in table 1). The true number of events is therefore impossible for readers to determine, as is the ability to investigate the effect on the results based on the full empirical dataset. Therefore, we used a subset with meta-analyses free of this type of ambiguous errors. We prespecified a 20% change of the magnitude or more of the effects as moderate impact and a 50% change or more as large impact. We also summarised the proportion of change on the direction of the effects and on the significance of the P value.
Descriptions of the different types of errors during the data extraction
Missing data would occur when the original data sources were not available for a few randomised controlled trials in which we were unable to verify data accuracy. For our sensitivity analysis, which investigated the robustness of the results, we removed these studies. We used Stata 15/SE for the data analysis. The estimation of the proportions was based on the meglm command under the Poisson function with the log link28; we set α=0.05 as the significance level. We performed the re-evaluation of the meta-analyses by the admetan command in Stata and verified by metafor command in R 3.5.1 software, and Excel 2013 was used for visualisation.
Patient and public involvement
As this was a technical paper to assess related methodology for data extraction errors of evidence synthesis practice and the impacts of these errors on the analysis, no patients or public members were involved, nor was funding available for the same reason.
Results
Overall, we screened 18 636 records, and initially identified 456 systematic reviews of adverse events.9 After a further screening of the full texts, 102 were excluded for having non-randomised studies of intervention and 153 were excluded for not having a pairwise meta-analysis, having fewer than five studies in all meta-analyses, or not reporting 2×2 table data used in meta-analyses (supplementary table 3). As such, 201 systematic reviews were included in the current study (fig 1).
Among the 201 systematic reviews, 156 referred to drug interventions and the other 45 were non-drug interventions (60% were surgical or device interventions). From the 201 systematic reviews, we identified 829 pairwise meta-analyses with at least five studies involving 10 386 randomised controlled trials. The data extraction error by the four data extractors ranged from 0.5% to 5.4% based on the double-checking process, which suggested that this study had high quality data extraction (supplementary table 1).
Among the 201 systematic reviews, based on the reporting information, 167 (83.1%) stated that they had two data extractors, 31 (15.4%) did not report such information, two (1%) cannot be judged owing to insufficient information, and only one (0.5%) reported that the data were extracted by one person. Fifty four (26.9%) systematic reviews reported a protocol that was developed in advance, whereas most (147, 73.1%) did not report whether they had a protocol. For those with protocols, 32 (59.3%) of 54 had a clear plan for data extraction and 22 (40.7%) outlined a potential solution for anticipant problems for data extraction. Sixty six (32.8%) systematic reviews used a standard data extraction form, while most (135, 67.2%) did not report this information. For the systematic reviews that used a standard extraction form, six (8.8%) piloted this process. No systematic reviews reported the information of whether the data extractor was trained or the expertise of the data extractor. Only seven (3.5%) of 201 systematic reviews documented the details of the data extraction process.
Reproducibility of the data extraction
For the reproducibility of the data used in these meta-analyses, at the study level, we could not reproduce 1762 (17.0%) of 10 386 studies with an extractor addressed proportion of 15.8%. At the meta-analysis level, 554 (66.8%) of 829 meta-analyses had at least one randomised controlled trial with data extraction errors, with an extractor addressed proportion of 65.5% (fig 2). For meta-analyses with data extraction errors in at least one study, the proportion of studies with data extraction errors within a meta-analysis ranged from 1.9% to 100%, with a median value of 20.6% (interquartile range 12.5-40.0; fig 2).
At the systematic review level, 171 (85.1%) of 201 systematic reviews had at least one meta-analysis with data extraction errors, with an extractor addressed proportion of 85.1% (fig 3). For systematic reviews with data extraction errors in at least one meta-analysis, the proportion of meta-analyses with data extraction errors within a systematic review ranged from 16.7% to 100.0%, with a median value of 100.0% (interquartile range 66.7-100; fig 3).
Data extraction errors at the systematic review level. Bar plot is based on studies with data extraction errors (n=171). Error rate within a systematic review is calculated by the number of meta-analyses with data extraction errors against the total number of meta-analyses within a systematic review
Based on the multivariable logistic regression, those systematic reviews that reported duplicated data extraction or were checked by another author (odds ratio 0.9, 95% confidence interval 0.3 to 2.5, P=0.83) and developed a protocol in advance (0.7, 0.3 to 1.6, P=0.38) did not show a difference in the odds of errors, but there might be a weak association of errors.
Empirical classification of errors
Based on the mechanism of the data extraction errors, we empirically classified these errors into five types: numerical error, ambiguous error, zero assumption error, mismatching error, and misidentification error. Table 1 provides the definitions of these five types of data extraction errors, with detailed examples.1718192021222324252627
Numerical error was the most prevalent data extraction error, which accounted for 867 (49.2%) of 1762 errors recorded in the studies (fig 4). The second most prevalent data extraction error was the ambiguous error, accounting for 526 (29.9%) errors. Notably, zero assumption errors accounted for as much as 221 (12.5%) errors. Misidentification accounted for 115 (6.5%) errors and mismatching errors accounted for 33 (1.9%) errors.
Subgroup analysis by the intervention type suggested that meta-analyses with drug interventions were more likely to have data extraction errors than those involving non-drug interventions: total error (19.9% v 8.9%; P<0.001), ambiguous error (6.1% v 2.4%; P<0.001), numerical error (9.4% v 5.4%; P<0.001), zero assumption error (2.6% v 0.9%; P<0.001), and misidentification errors error (1.5% v 0.1%; P<0.001; supplementary fig 3). Although mismatching errors showed the same pattern, the data were not significantly different (0.4% v 0.2%; P=0.09).
Impact of data extraction errors on the results
After removing meta-analyses with ambiguous errors and without errors, 288 meta-analyses could be used to investigate the impact of data extraction errors on the results (supplementary table 4). Among them, 39 had two or more types of errors (mixed), and 249 had only one type of error (single). For the 249 meta-analyses, 200 had numerical errors, 25 had zero assumption errors, 16 had misidentification errors, and eight had mismatching errors. Because of the limited sample size of each subtype, we only summarised the total impact and the effect grouped by the number of types (that is, single type of error or mixed type of errors).
In total, in terms of the magnitude of the effect, when using corrected data for the 288 meta-analyses, 151 (52.4%) had decreased effects, whereas 137 (47.6%) had increased effects; 37 (12.8%) meta-analyses had moderate changes (with ≥20% changes), and 13 (4.5%) had large changes (with ≥50% changes) in the effect estimates (fig 5). For those 37 studies with moderate changes, the effects in 26 (70.2%) increased, whereas those in 11 (29.7%) decreased when using corrected data. For those 13 studies with large changes, nine (69.2%) showed increased effects, whereas four (30.8%) showed decreased effects. Ten (3.5%) of the 288 meta-analyses had changes in the direction of the effect, and 19 (6.6%) of the 288 meta-analyses changed the significance of the P value. For those studies that had changes in the direction, two (20.0%) of 10 changed from beneficial to harmful effects, and eight (80.0%) of the 10 changed from harmful to beneficial effects. For studies that changed in significance, 10 (52.6%) of 19 changed from non-significance to significance, and nine (47.4%) of 19 changed from significance to non-significance. Some examples are presented in table 2. Studies with two or more types of errors had higher proportions of moderate (28.2% v 10.4%, P=0.002) and large changes (12.8% v 3.2%, P=0.01; fig 5) than did with only a single error.
Examples of changes in the effects and significance when using corrected data
Sensitivity analysis
For 318 (3.1%) of 10 386 studies in the total dataset, we could not obtain full texts or had no access to the original data source to verify data accuracy. After treating them as missing values and removing them from the analyses, no changes were obvious in the proportions of data extraction errors: 16.2% for the study level, 65.7% for the meta-analysis level, and 85.1% for the systematic review level (addressed by extractor clustering effects).
Discussion
Principal findings
We investigated the reproducibility of the data extraction of 829 pairwise meta-analyses within 201 systematic reviews of safety outcomes by repeating the data extraction from all the included studies. Our results suggested that as much as 85% of the systematic reviews had data extraction errors in at least one meta-analysis. From the point of meta-analysis level, as many as 67% of the meta-analyses had at least one study with data extraction error. Our findings support the seriousness of the findings from the survey conducted by Nature regarding reproducibility of basic science research (70%).1 At the systematic review level, the problem is even more serious.
Our subgroup analysis showed that data for the safety outcomes of drug interventions had a higher proportion of extraction error (19.9%) than did data for non-drug interventions (8.9%). One important reason could be that safety outcomes of different types of interventions vary considerably (supplementary fig 1). For non-drug interventions, most interventions were surgical or a device, where safety outcomes might be easier to define. For example, a common safety outcome in surgical intervention is bleeding during surgery, whereas a common outcome of drug interventions is liver toxicity, which might be more complex to define and measure. Additionally, the reporting of adverse events in surgical interventions heavily relies on the surgical staff, whereas for adverse events of a drug, patients might also participate in the reporting process. Selective reporting could exist for adverse events of surgical interventions without patients’ participation,29 and mild but complex adverse events (eg, muscular pain) might be neglected and further make reported adverse events appear more straightforward.
We classified data extraction errors into five types based on the mechanism. Based on this classification, we further found that numerical errors, ambiguous errors, and zero assumption errors accounted for 91% of the total errors. The classification and related findings are important because these data provide a theoretical basis for researchers to develop implementation guidelines and help systematic review authors to reduce the frequency of errors during the data extraction process. Another important reason for data extraction errors might be the poor reporting of adverse events in randomised controlled trials, which have varying terminology, poorly defined categories, and diverse data sources.303132 If trials did not clearly define an adverse outcome and report it transparently, then systematic review authors would face difficulties during data extraction and the process would be prone to errors, especially with regard to the ambiguous types. We believe that with proper implementation guidance and more explicit trial reporting guidelines for adverse events, these errors can substantially be reduced.
The classification also provides a theoretical basis for methodologists to investigate the potential impact of different types of data extraction errors on the results. The impact of different types of errors on the results could vary. For example, the zero assumption error is expected to push the final effect towards the null when related studies have balanced sample sizes in two arms.33 The mismatching error has a similar effect because the error pushes the effect towards the opposite direction. By contrast, the direction of the effect is difficult to predict in the other three types of errors. In our empirical data, because of the small number of meta-analyses in each category, we were unable to investigate the impact of each single type of error on the results. One of the most important reasons is that many meta-analyses have ambiguous errors. Nevertheless, we were able to compare the effect of multiple error types against a single error type for meta-analyses. Our results suggested that meta-analyses with multiple types of data extraction errors were prone to be affected. Because different methods can vary on this assumption (eg, two-stage methods with odds ratios assume that double-zero studies are non-informative9), the use of different synthesis methods and effect estimates might have different impacts.3435 The impact of data extraction errors on the results is expected to be thoroughly investigated by simulation research.
Strengths and limitations
This large empirical study investigates the reproducibility of the data extraction of systematic reviews of adverse events and its impact on the results of related meta-analyses. The findings of our study pose a serious warning to the community that much progress is needed to achieve high quality, evidence-based practice. We are confident that the results of our findings are reliable because the data have been through five rounds of cross-checking within our tightly structured collaborative team. Additionally, this study is the first time that data extraction errors were defined based on their mechanism, which we think will benefit future methodological research in this area.
However, some limitations are still present. Firstly, owing to the large amount of work, data collection was divided into four portions, and each portion was conducted by a separate author. Although all authors undertook pilot training in advance, their judgments might still differ. Nevertheless, our analysis used the generalised linear mixed model, which accounted for the potential clustering effect by different extractors, of which the findings suggested no obvious impact on the results. Secondly, our study covered only systematic reviews published in the five year period from 2015 to 2020; therefore, the validity of the data extraction in earlier studies is unclear. Whether this issue has deteriorated or improved over time could not be assessed. Thirdly, a small proportion of studies could not have reproducibility checked, and these studies were treated as if no data extraction errors existed, which could lead to a slight underestimation of data extraction error overall.36
Furthermore, we only focused on systematic reviews of randomised controlled trials and did not consider observational studies. Because the sample sizes of randomised controlled trials tend to be small, the impact might be exacerbated. Finally, poor reporting has been commonly investigated in literature3738; owing to the limited information of the data extraction process reported by review authors, we could not fully investigate the association between good practice recommendations and the likelihood of data extraction. For the same reason, the association among duplicated data extraction, development of a protocol in advance, and data extraction errors should be interpreted with caution. Further studies based on randomised controlled design might be helpful. However, we believe these limitations have little impact on our main results and conclusions.
Conclusions
Systematic reviews of adverse events face serious issues in terms of the reproducibility of their data extraction. Prevalence of data extraction errors is high among these systematic reviews and these errors could lead to the changing of the conclusions and further mislead the healthcare practice. A series of expanded reproducibility studies on other types of meta-analyses might be useful for further evidence-based practice. Additionally, implementation guidelines on data extraction for systematic reviews are urgently required to help future review authors improve the validity of their findings.
What is already known on this topic
-
In evidence synthesis practice, data extraction is an important step and prone to errors, because raw data are transferred from the original studies into the meta-analysis
-
Data extraction errors in systematic reviews occur frequently in the literature, although these errors generally have a minor effect on the results
-
However, this conclusion is based on systematic reviews of continuous outcomes, and might not apply to binary outcomes of adverse events
What this study adds
-
In a large-scale reproducibility investigation of 201 systematic reviews of adverse events with 829 pairwise meta-analyses, data extraction errors frequently occurred for binary outcomes of adverse events
-
These errors could be grouped into five categories based on the mechanism: numerical error, ambiguous error, zero assumption error, mismatching error, and misidentification error
-
The errors can lead to changes in the conclusions of the findings, and meta-analyses that had two or more types of errors were more susceptible to these changes